The Advanced Imaging and Informatics for Radiation Therapy (AIRT) Lab's research is focused on the development of novel imaging and beam delivery techniques and new machine learning algorithms to improve the efficacy of radiation therapy. We are interested in medical image processing, image reconstruction, signal processing, treatment outcome prediction, image-guided radiation therapy (IGRT), and adaptive radiation therapy (ART). We are working on both software and hardware approaches to enhance the quality of different medical imaging modalities (including CT, CBCT, PET, and MRI) for their quantitative applications in image-guided and adaptive radiation therapy. We have also developed a number of machine-learning algorithms for image reconstruction, medical imaging analysis, and treatment outcome prediction.
The topics we are currently working on include:
- Low-dose CT and cone-beam CT
- 4D lung cone-beam CT imaging
- 4D liver cone-beam CT imaging and tumor localization
- Quantitative imaging for adaptive radiation therapy
- 4D MRI reconstruction
- Machine learning for treatment outcome prediction and classification
Our research has been supported by NIH National Institute of Biomedical Imaging and Bioengineering (R01 EB020366, R01 EB027898), American Cancer Society (RSG-13-326-01-CCE and ACS-IRG-02-196), Cancer Prevention and Research Institute of Texas (RP130109, RP110562, and RP160661), and Elekta Ltd.
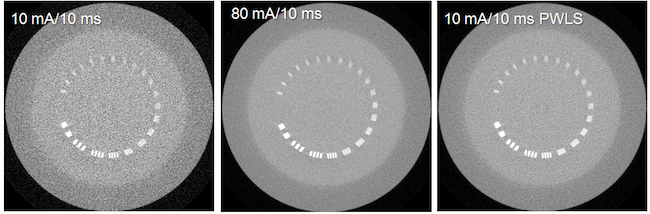
There is growing concern on the radiation dose delivered to patient during CT or cone-beam CT (CBCT) procedure, especially for pediatric patients, screening, or repeated acquisitions for CBCT-guided radiation therapy over a whole treatment course. We have developed various strategies to enhance image quality of low-dose CT and CBCT.
Our research focuses on:
- Modeling the statistical properties of noise in low-dose CT/CBCT data.
- Developing and optimizing statistics-based projection restoration and iterative image reconstruction algorithms. We are currently evaluating these algorithms on clinical patient images.
Related publications
- J. Wang, T. Li, H. Lu, and Z. Liang, “Penalized weighted least-squares approach to sinogram noise reduction and image reconstruction for low-dose X-ray computed tomography,” IEEE Trans. on Medical Imaging, vol. 25, pp. 1272-1283, 2006.
- J. Wang, T. Li, and L. Xing, “Iterative image reconstruction for CBCT using edge-preserving prior,” Medical Physics, vol. 36, pp. 252-260, 2009.
- L. Ouyang, T. Solberg, and J. Wang, “Effects of the penalty to penalized weighted least-squares image reconstruction for low-dose CBCT,” Physics in Medicine and Biology, vol. 56, pp. 5535-5552, 2011.
- J. Wang, H. Guan and T. Solberg, “Inverse determination of the penalty parameter in penalized weighted least-squares algorithm for noise reduction of low-dose CBCT,” Medical Physics, vol. 38, pp. 4066-4072, 2011.
- L. Ouyang, T. Solberg, and J. Wang, “Noise Reduction in Low-Dose Cone Beam CT by Incorporating Prior Volumetric Image Information,” Medical Physics, vol. 39, pp. 2569-2577, 2012.
- J. Wang, J. Robar, and H. Guan, “Noise Suppression in Reconstruction Low-Z Target MV CBCT Images,” Medical Physics, vol. 39, pp 5111-5117, 2012.
- H. Zhang, L. Ouyang, J. Ma, J. Huang, W. Chen, and J. Wang, “Noise Correlation in CBCT Projection Data and its Application for Noise Reduction in Low-dose CBCT”, Medical Physics, vol. 41, 031906, 2014
- H. Zhang, L. Ouyang, J. Huang, J. Ma, W. Chen, and J. Wang, "Few-view cone-Beam CT Reconstruction with Deformed Prior Image", Medical Physics, vol. 41, 121905, 2014
- T. Sun, N. Sun, J. Wang*, and S. Tan, "Iterative CBCT reconstruction using hessian penalty", Physics in Medicine and Biology, vol. 60, pp.1965-1987, 2015 (*co-corresponding author)
Respiration-induced tumor motion causes uncertainties and challenges when radiation is used to treat tumors in thoracic or upper abdominal regions. We are interested in developing novel strategies to manage tumor motion in radiation therapy. One of our recent research interests is on the development of high-quality four dimensional cone-beam CT (4D-CBCT). We developed a new 4D image reconstruction strategy that can perform simultaneous motion estimation and image reconstruction (SMEIR).
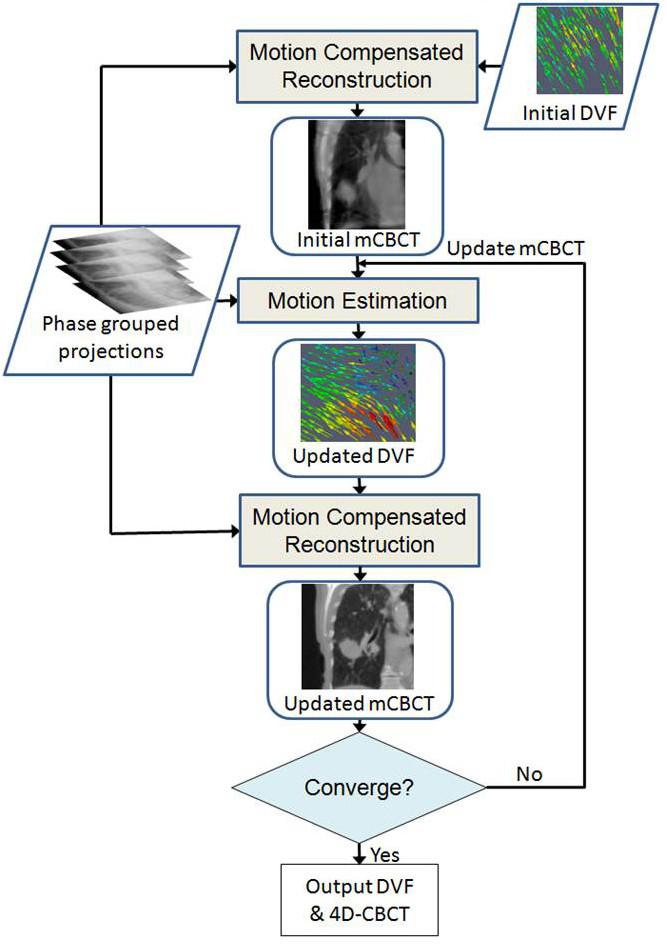
- J. Wang, and X. Gu, "High quality four dimensional cone-beam CT by deforming prior images," Physics in Medicine and Biology, vol. 58, pp. 231-246, 2013.
- J. Wang and X. Gu, "Simultaneous motion estimation and image reconstruction (SMEIR) for 4D cone-beam CT", Medical Physics, vol. 40, pp. 101912, 2013
- J. Dang, X. Gu , T. Pan and J. Wang, "A pilot clinical evaluation of a 4D-CBCT scheme based on Simultaneous Motion Estimation and Image Reconstruction", Int J Radiat Oncol Biol Phys, vol. 91, pp. 410-418, 2015
Liver tumor imaging and localization remains a challenge task for modern image-guided radiation therapy. The liver tumor is usually of low contrast in x-ray imaging, rendering it hardly discernable from surrounding normal liver tissues. Correspondingly, a large treatment margin needs to be added beyond the actual extent of the liver tumor, to account for the uncertainties of liver tumor localization for radiation therapy, to ensure sufficient tumor coverage by radiation beams. Such a practice unfortunately also adds unnecessary dose to normal liver tissues, increases liver toxicity, and potentially limits the efficacy of radiation therapy, especially those using large fractional doses like SBRT. We developed an advanced reconstruction technique combining iterative reconstruction with deformable registration and biomechanical modeling to allow automatic, accurate 4D liver tumor imaging and localization using non-contrast CBCT projections (Bio-4DCBCT). Bio-4DCBCT allows substantial tumor localization accuracy improvement to within 2 mm, enabling accurate and safety radiation therapy to improve patient outcomes.
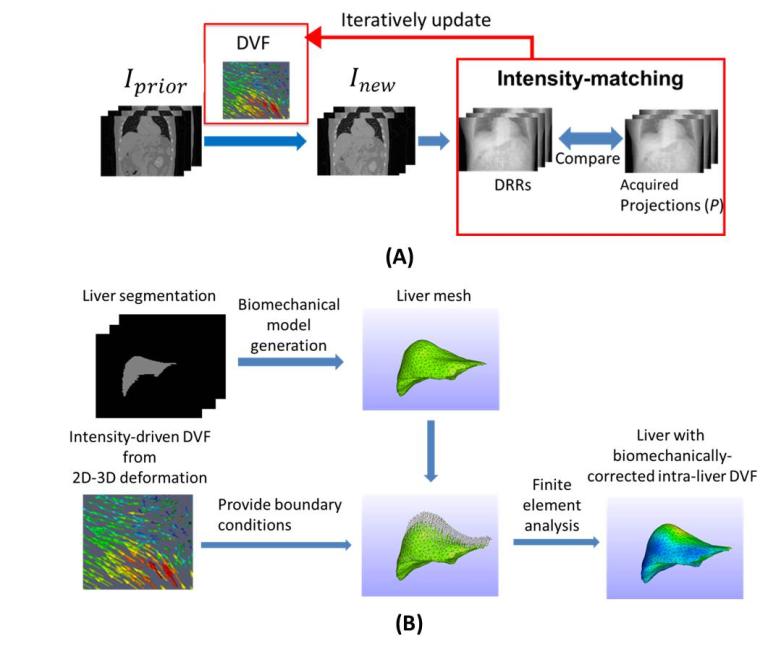
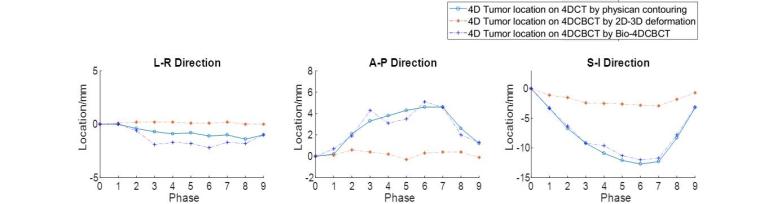
- Y. Zhang, M. Folkert, B. Li, X. Huang, J. Meyer, T. Chiu, P. Lee, J. Tehrani, J. Cai, D. Parsons, X. Jia, and J. Wang, “4D Liver Tumor Localization using Cone-Beam Projections and a Biomechanical Model", Radiotherapy & Oncology 133, 183-192 (2019).
- Y. Zhang, M. R. Folkert, X. Huang, J. Meyer, J. N. Tehrani, L. Ren, and J. Wang, "Enhancing Liver Tumor Localization Accuracy by Prior-Knowledge-Guided Motion Modeling and A Biomechanical Model ", Quantitative Imaging in Medicine and Surgery 9(7), 1337-1349 (2019).
- Y. Zhang, X. Huang, and J. Wang, "Advanced 4D-CBCT reconstruction by combining motion estimation, motion-compensated reconstruction, biomechanical modeling and deep learning", Visual Computing for Industry, Biomedicine, and Art, 2, 23 (2019).
- Y. Zhang, J.N. Tehrani, and J. Wang, "A biomechanical modeling guided CBCT estimation technique ", IEEE Transactions on Medical Imaging 36, 641-652 (2017).
On-board imaging can provide updated patient information at the treatment position thus can be potentially used for adaptive radiation therapy. On-board imaging is often degraded by various artifacts, which makes it challenging for quantitative applications. We are interested in the development of quantitative imaging techniques for their applications in image-guided and adaptive radiation therapy. One of our recent research focuses is on the development and evaluation of a quantitative CBCT technique.
We developed a moving-blocker-based approach that is able to suppress the scatter-related artifacts, reduce the imaging dose, and simultaneously obtain complete volumetric information within the field of view using a single CBCT scan.
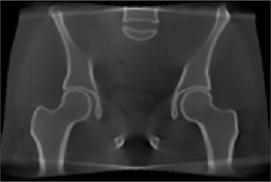
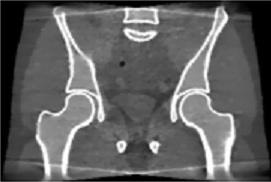
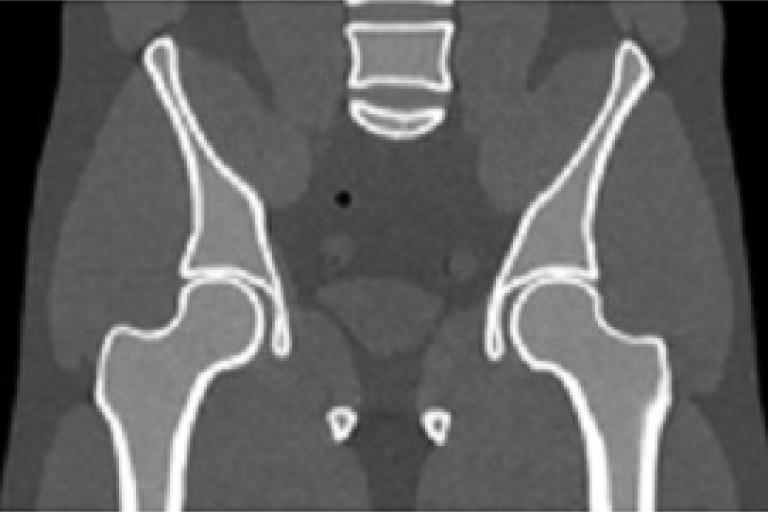
- J. Wang, W. Mao, and T. Solberg, "Scatter correction for cone-beam computed tomography using one-dimensional moving blocker strips: a preliminary study", Medical Physics, vol. 37, pp. 5792-5800, 2010.
- L. Ouyang, K. Song, and J. Wang, "A moving blocker system for cone-beam computed tomography scatter correction", Medical Physics, vol. 40, pp. 071903, 2013
- L. Ouyang, P. Lee, and J. Wang, "A moving-blocker-based strategy for simultaneous megavoltage and kilovoltage scatter correction in cone-beam computed tomography image acquired during volumetric modulated arc therapy", Radiotherapy and Oncology, to appear, 2015
4D volumetric MRI has shown clear advantages in radiotherapy for soft-tissue tumor delineation and mobile target localization/tracking with zero ionization radiation hazard. However, 4D volumetric MRI has not been fully established in current clinical practice, mostly due to the physical constraints that lead to the challenging task of achieving high temporal resolution imaging while maintaining adequate spatial resolution. The popular retrospective sorting approach used in 4D-MRI generation leads to k-space under-sampling and causes aliasing artifacts in the resulting MR images. This project proposes to develop a simultaneous k-space-driven motion estimation and compensation (SK-MEC) method for 4D-MRI reconstruction. SK-MEC explores the feasibility of combining k-space-driven inter-respiratory-phase motion estimation with motion-compensated reconstruction for 4D-MRI reconstruction from under-sampled k-space data. By motion-compensated reconstruction, SK-MEC uses the entire k-space dataset and a motion model to reconstruct a reference (respiratory) phase MRI, which substantially reduces the aliasing artifacts from under-sampling. By k-space-driven motion-estimation, SK-MEC directly solves the motion fields between the reference (respiratory) phase and the other (respiratory) phases through k-space data matching, thus eliminating the uncertainties from solving motion by registering two reconstructed MRIs of varying image quality and incoherent aliasing artifacts. Our preliminary study on digitally simulated k-space data has yielded encouraging results for the proposed technique. In this project we propose to develop and optimize the SK-MEC method using raw k-space data of phantoms and in vivo data from clinical/research Philips MRI scanners. We will initiate the study using raw k-space data of static phantoms (without motion), to evaluate the general integrity of our reconstruction algorithm. After the initial step, we will acquire mobile phantom raw k-space data using the same scanner, to evaluate, optimize, and test the performance of the proposed SK-MEC algorithm to generate 4D-MRI images. We will evaluate different k-space sparsity strategy, and quantify the acceleration potential, and the accuracy of SK-MEC in 4D-MRI acquisition. We will evaluate and choose a corresponding pulse-sequence that works best with our algorithm, with potential modifications and developments to currently available pulse sequences. In the end, an in-vivo dataset will be used to further optimize, validate, and evaluate the SK-MEC algorithm. The successful completion of the study will pave the way for a further MRI-guided radiation therapy study which aims to reduce the treatment damages to normal liver tissues and improve the efficacy of liver tumor treatment.
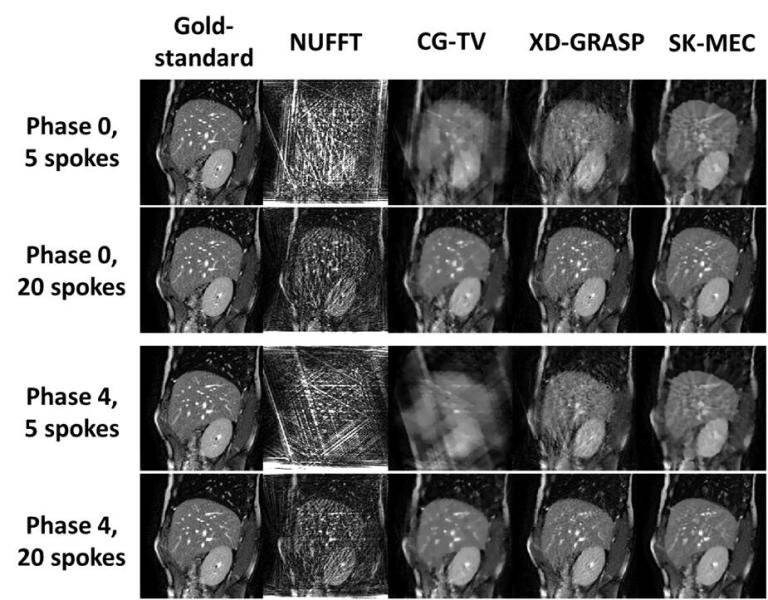
Figure 1. Reconstructed image comparison between different techniques, using k-space data simulated from available MR images. Phase X: respiratory phase X. X spokes: the number (X) of spokes of each respiratory phase used in 4D-MRI reconstruction. A golden-angle, radial sampling strategy was employed. NUFFT: non-uniform fast Fourier transform algorithm. CG-TV: conjugate-gradient algorithm with total variation regularization. XD-GRASP: eXtra dimensional golden-angle radial sparse parallel algorithm, a published advanced reconstruction technique for 4D-MRI. The SK-MEC technique offers superior image quality compared to the other techniques, even for severely under-sampled cases (5 spokes per respiratory phase).
- Y. Zhang, Z. Iqbal, C. Shen, C. Wang, S. Jiang, J. Wang, “ Dynamic MRI Reconstruction Using Simultaneous K-Space-Driven Motion Estimation and Compensation (SK-MEC) ”, AAPM Annual Meeting, 2019
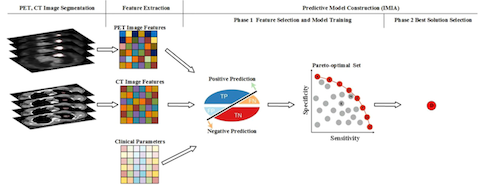
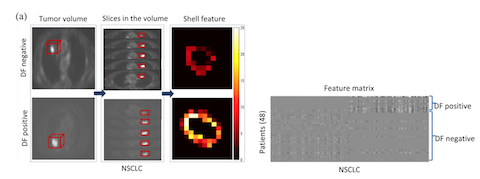
We are developing new machine learning methods for image reconstruction, classification and treatment outcome prediction. Early prediction of treatment outcome is important in clinical decision for patient management in radiotherapy. We have developed a number of new radiomics features and different machine learning algorithms such as kernelled support tensor vector machine for distant failure prediction of NSCLC and cervical cancer patients after radiation therapy. We have also developed a multi-modality radiomics model coupled with an immune based optimization strategy to improve the performance of these prediction models.
- Zhou, M. Folkert, N. Cannon, P. Iyengar, K. Westover, Y. Zhang, H. Choy, R. Timmerman, S. Jiang, J. Yan, X-J Xie, and J. Wang, Predicting distant failure in early stage NSCLC treated with SBRT using clinical parameters, Radiotherapy and Oncology, vol. 119, pp. 501-504, 2016
- Zhou, M. Folkert, P. Iyengar, K. Westover, Y. Zhang, H. Choy, R. Timmerman, S. Jiang, and J. Wang, “Multi-objective radiomics model for predicting distant failure in lung SBRT", Physics in Medicine and Biology, vol. 62, pp. 4460-4478, 2017
- Hao, Z. Zhou, S. Li, G. Maquilan, M. Folkert, P. Iyengar, K. Westover, K. Albuquerque, F. Liu, H. Choy, R. Timmerman, L. Yang, and J. Wang, Shell: a new radiomics descriptor for predicting distant failure after radiotherapy in non-small cell lung cancer and cervical cancer, Physics in Medicine and Biology, vol. 63, 095007 (17pp), 2018
- Li, B. Li, Z. Zhou, N. Yang, H. Hao, M. Folkert, P. Iyengar, K. Westover, H. Choy, R. Timmerman, S. Jiang, and J. Wang, A pilot study using kernelled support tensor machine for distant failure prediction in lung SBRT, Medical Imaging Analysis, vol. 50, pp.106-116, 2018
- Chen, K. Xiang, Z. Gong, J. Wang*, and S. Tan, Statistical Iterative CBCT Reconstruction Based on Neural Network, IEEE Trans. on Medical Imaging, vol. 37, pp. 1511-1521, 2018 (*co-corresponding author), Code for this work.
-
Z. Zhou, S. Li, G. Qin, M. Folkert, S. Jiang, and J. Wang, Automatic multi-objective based feature selection for classification, (arXiv version, and the correspond code can be downloaded.)