Drug discovery: an industry in crisis
Drug discovery is in a crisis: the cost of a new drug is estimated to be more than $2B after having doubled every nine years since the 1950s (Scannell et al., 2012). Furthermore, oncology is the therapeutic area with the lowest likelihood of approval from phase I trials at 5.1%, which compares to an average of 9.6% for all indications, or 19.1% for infectious diseases (Mullard, 2016). Experts point out the prevalence of suboptimal preclinical strategies as one potential reason for low success rates in oncology (Hutchinson and Kirk, 2011). Specifically, preclinical cancer drug discovery relies on screening monolayer, monoclonal cancer cell lines on glass/plastic surfaces, typically with a single viability readout (Barretina et al., 2012; Basu et al., 2013; Iorio et al., 2016; Seashore-Ludlow et al., 2015; Yang et al., 2013). These studies have provided many valuable insights into cancer cell-intrinsic pathways. However, they have two limitations. The first is that the chemical space is for all practical purposes virtually infinite (Lipinski and Hopkins, 2004) therefore brute-force screening will always barely scratch the surface, no matter the improvements in robotics or synthesis. The second limitation is that cancer is a disease that involves more than just the cancer cells—the tumor microenvironment also has a significant role to play (Tabassum and Polyak, 2015). Functional assays use more involved assay designs (Friedman et al., 2015) yet due to their higher complexity they typically have lower throughput. Thus it is not easy to use a functional assay for a high throughput screen which requires millions of experiments (Macarron et al., 2011). Instead, functional assays are proposed as clinical drug response diagnostics (Majumder et al., 2015). We hypothesize that we can use machine learning to select experiments and thus enable the use of limited throughput functional assays for drug discovery.
A convergence of technologies: machine learning, single-cell RNA-sequencing, and big data
We live in a world where the revolutionary promise of artificial intelligence (2017), single-cell RNA-sequencing technology (Liu and Trapnell, 2016), and big data (Luo et al., 2016) are well recognized. Overlaps between these trends are also appreciated (Yu and Lin, 2016). Beyond the hype, we believe there is a genuine opportunity to put these three important technological trends together to create a clinically relevant cancer drug discovery pipeline. Below, we outline specifically how we plan to benefit from each of these technologies and finally lay out the integrated vision.
Machine Learning
Machine learning (ML) is the field of artificial intelligence (AI) that aims to build systems that improve with experience (Jordan and Mitchell, 2015). In recent years, there has been a wave of excitement about the potential of ML and AI in various disciplines, including biomedicine (Russell, 2017; Stajic et al., 2015). The investigation of the role of ML in drug discovery predates the current wave of excitement. Our work (Cobanoglu et al., 2013; Wise and Cobanoglu, 2016) and the work of many other groups (Kangas et al., 2014; Kearnes et al., 2016; Lavecchia, 2015; Murphy, 2011; Naik et al., 2016; Reker and Schneider, 2015; Riniker et al., 2014; Warmuth et al., 2003) have shown that machine learning can significantly increase the efficiency of drug discovery by intelligently guiding experimentation. These efforts from multiple labs naturally span different goals and designs. However, the central theme is that machine learning can intelligently select the next experiment to conduct based on available data, and do this more effectively than arbitrarily screening some chemical subspace.
Single-cell RNA-sequencing
The tumor microenvironment is complex with multiple cell types present: tumor-infiltrating lymphocytes (TILs), stromal cells (such as cancer-associated fibroblasts), and malignant cells. Furthermore, these cell populations are heterogeneous in and of themselves (Patel et al., 2014; Tirosh et al., 2016). Bulk RNA-sequencing of the tumor is unfortunately only able to provide a mixed signal and deconvolution is only approximate (Li et al., 2017; Newman et al., 2015; Zhu et al., 2016). Single-cell RNA-sequencing (scRNA-seq) has the potential to revolutionize the study of cancer, by providing data about the state of each cell separately. There exist many computational tools for the analysis of scRNA-seq (Perkel, 2017) and we are also working on addressing some perceived limitations with existing tools.
Big data biology
We live in an age where The Economist declares “the world’s most valuable resource is no longer oil, but data” (May 6th, 2017). In line with these global trends, science has also transformed. Currently, there exist many different datasets with publicly available data (at least for non-profit biomedical researchers) and easy-to-use formats. We plan to make ample use of these resources.
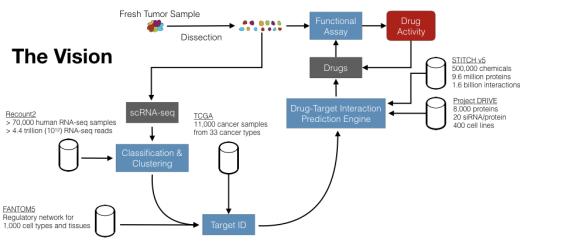
We think that the Recount2 database (Collado-Torres et al., 2017) is a valuable resource for accessing bulk RNA-sequencing data produced over the last decade. Specifically, we plan to utilize purified bulk RNA-seq data when analyzing scRNA-seq data. The FANTOM5 (Noguchi et al., 2017) provides us with CAGE-seq profiles from almost every human cell & tissue context of interest. This dataset has been used to derive context-specific TF-gene regulation networks (Marbach et al., 2016). We utilize these networks in our regulation-based differential analysis of gene expression data. The TCGA provides ample data, albeit in bulk RNA-seq form, about different types of cancer (National Cancer Institute). Project DRIVE (McDonald et al., 2017) and other similar initiatives provide insight into cancer vulnerabilities through the knockdown of genes. Despite the limitations of monoclonal cell culture listed above, these resources provide valuable inference and testing grounds for novel algorithm development. In parallel, the STITCH database, currently in its fifth iteration (Szklarczyk et al., 2016), ‘stitches’ together many different datasets to create a single unified list of all known drug-target interactions. This is a gargantuan compendium of data, with about 1.6 billion interactions listed between close to 10 million proteins and half a million chemicals. We intend to utilize this dataset when building our drug-target interaction prediction engine.
We think that currently, we are the confluence of three individually powerful trends, that hold the potential to deliver more than the sum of their parts. We have a vision for converging them in our lab to deliver, eventually, a human tumor-driven cancer drug discovery pipeline. We hope that this preclinical pipeline can increase the later stage clinical success.
References
Barretina, J., Caponigro, G., Stransky, N., Venkatesan, K., Margolin, A.A., Kim, S., Wilson, C.J., Lehár, J., Kryukov, G.V., Sonkin, D., et al. (2012). The Cancer Cell Line Encyclopedia enables predictive modelling of anticancer drug sensitivity. Nature 483, 603–607.
Basu, A., Bodycombe, N.E., Cheah, J.H., Price, E.V., Liu, K., Schaefer, G.I., Ebright, R.Y., Stewart, M.L., Ito, D., Wang, S., et al. (2013). An interactive resource to identify cancer genetic and lineage dependencies targeted by small molecules. Cell 154, 1151–1161.
Cobanoglu, M.C., Liu, C., Hu, F., Oltvai, Z.N., and Bahar, I. (2013). Predicting drug-target interactions using probabilistic matrix factorization. J. Chem. Inf. Model. 53, 3399–3409.
Collado-Torres, L., Nellore, A., Kammers, K., Ellis, S.E., Taub, M.A., Hansen, K.D., Jaffe, A.E., Langmead, B., and Leek, J.T. (2017). Reproducible RNA-seq analysis using recount2. Nat. Biotechnol. 35, 319–321.
Friedman, A.A., Letai, A., Fisher, D.E., and Flaherty, K.T. (2015). Precision medicine for cancer with next-generation functional diagnostics. Nat. Rev. Cancer 15, 747–756.
Hutchinson, L., and Kirk, R. (2011). High drug attrition rates—where are we going wrong? Nat. Rev. Clin. Oncol. 8, 189–190.
Iorio, F., Knijnenburg, T.A., Vis, D.J., Bignell, G.R., Menden, M.P., Schubert, M., Aben, N., Gonçalves, E., Barthorpe, S., Lightfoot, H., et al. (2016). A Landscape of Pharmacogenomic Interactions in Cancer. Cell 166, 740–754.
Jordan, M.I., and Mitchell, T.M. (2015). Machine learning: Trends, perspectives, and prospects. Science 349, 255–260.
Kangas, J.D., Naik, A.W., and Murphy, R.F. (2014). Efficient discovery of responses of proteins to compounds using active learning. BMC Bioinformatics 15, 143.
Kearnes, S., McCloskey, K., Berndl, M., Pande, V., and Riley, P. (2016). Molecular graph convolutions: moving beyond fingerprints. J. Comput. Aided Mol. Des.
Lavecchia, A. (2015). Machine-learning approaches in drug discovery: methods and applications. Drug Discov. Today 20, 318–331.
Li, T., Fan, J., Wang, B., Traugh, N., Chen, Q., Liu, J.S., Li, B., and Liu, X.S. (2017). TIMER: A Web Server for Comprehensive Analysis of Tumor-Infiltrating Immune Cells. Cancer Res. 77, e108–e110.
Lipinski, C., and Hopkins, A. (2004). Navigating chemical space for biology and medicine. Nature 432, nature03193.
Liu, S., and Trapnell, C. (2016). Single-cell transcriptome sequencing: recent advances and remaining challenges. F1000Res. 5.
Luo, J., Wu, M., Gopukumar, D., and Zhao, Y. (2016). Big Data Application in Biomedical Research and Health Care: A Literature Review. Biomed. Inform. Insights 8, 1–10.
Macarron, R., Banks, M.N., Bojanic, D., Burns, D.J., Cirovic, D.A., Garyantes, T., Green, D.V.S., Hertzberg, R.P., Janzen, W.P., Paslay, J.W., et al. (2011). Impact of high-throughput screening in biomedical research. Nat. Rev. Drug Discov. 10, 188–195.
Majumder, B., Baraneedharan, U., Thiyagarajan, S., Radhakrishnan, P., Narasimhan, H., Dhandapani, M., Brijwani, N., Pinto, D.D., Prasath, A., Shanthappa, B.U., et al. (2015). Predicting clinical response to anticancer drugs using an ex vivo platform that captures tumour heterogeneity. Nat. Commun. 6, 6169.
Marbach, D., Lamparter, D., Quon, G., Kellis, M., Kutalik, Z., and Bergmann, S. (2016). Tissue-specific regulatory circuits reveal variable modular perturbations across complex diseases. Nat. Methods 13, 366–370.
McDonald, E.R., 3rd, de Weck, A., Schlabach, M.R., Billy, E., Mavrakis, K.J., Hoffman, G.R., Belur, D., Castelletti, D., Frias, E., Gampa, K., et al. (2017). Project DRIVE: A Compendium of Cancer Dependencies and Synthetic Lethal Relationships Uncovered by Large-Scale, Deep RNAi Screening. Cell 170, 577–592.e10.
Mullard, A. (2016). Parsing clinical success rates. Nat. Rev. Drug Discov. 15, 447.
Murphy, R.F. (2011). An active role for machine learning in drug development. Nat. Chem. Biol. 7, 327–330.
Naik, A.W., Kangas, J.D., Sullivan, D.P., and Murphy, R.F. (2016). Active machine learning-driven experimentation to determine compound effects on protein patterns. Elife 5.
National Cancer Institute Program Overview.
Newman, A.M., Liu, C.L., Green, M.R., Gentles, A.J., Feng, W., Xu, Y., Hoang, C.D., Diehn, M., and Alizadeh, A.A. (2015). Robust enumeration of cell subsets from tissue expression profiles. Nat. Methods 12, 453–457.
Noguchi, S., Arakawa, T., Fukuda, S., Furuno, M., Hasegawa, A., Hori, F., Ishikawa-Kato, S., Kaida, K., Kaiho, A., Kanamori-Katayama, M., et al. (2017). FANTOM5 CAGE profiles of human and mouse samples. Sci Data 4, 170112.
Patel, A.P., Tirosh, I., Trombetta, J.J., Shalek, A.K., Gillespie, S.M., Wakimoto, H., Cahill, D.P., Nahed, B.V., Curry, W.T., Martuza, R.L., et al. (2014). Single-cell RNA-seq highlights intratumoral heterogeneity in primary glioblastoma. Science 344, 1396–1401.
Perkel, J.M. (2017). Single-cell sequencing made simple. Nature 547, 125–126.
Reker, D., and Schneider, G. (2015). Active-learning strategies in computer-assisted drug discovery. Drug Discov. Today 20, 458–465.
Riniker, S., Wang, Y., Jenkins, J.L., and Landrum, G.A. (2014). Using information from historical high-throughput screens to predict active compounds. J. Chem. Inf. Model. 54, 1880–1891.
Russell, S. (2017). Artificial intelligence: The future is superintelligent. Nature 548, 520–521.
Scannell, J.W., Blanckley, A., Boldon, H., and Warrington, B. (2012). Diagnosing the decline in pharmaceutical R&D efficiency. Nat. Rev. Drug Discov. 11, 191–200.
Seashore-Ludlow, B., Rees, M.G., Cheah, J.H., Cokol, M., Price, E.V., Coletti, M.E., Jones, V., Bodycombe, N.E., Soule, C.K., Gould, J., et al. (2015). Harnessing Connectivity in a Large-Scale Small-Molecule Sensitivity Dataset. Cancer Discov. 5, 1210–1223.
Stajic, J., Stone, R., Chin, G., and Wible, B. (2015). Artificial intelligence. Rise of the Machines. Science 349, 248–249.
Szklarczyk, D., Santos, A., von Mering, C., Jensen, L.J., Bork, P., and Kuhn, M. (2016). STITCH 5: augmenting protein-chemical interaction networks with tissue and affinity data. Nucleic Acids Res. 44, D380–D384.
Tabassum, D.P., and Polyak, K. (2015). Tumorigenesis: it takes a village. Nat. Rev. Cancer 15, 473–483.
Tirosh, I., Izar, B., Prakadan, S.M., Wadsworth, M.H., Treacy, D., Trombetta, J.J., Rotem, A., Rodman, C., Lian, C., Murphy, G., et al. (2016). Dissecting the multicellular ecosystem of metastatic melanoma by single-cell RNA-seq. Science 352, 189–196.
Warmuth, M.K., Liao, J., Ratsch, G., Mathieson, M., Putta, S., and Lemmen, C. (2003). Active Learning with Support Vector Machines in the Drug Discovery Process. J. Chem. Inf. Comput. Sci. 43, 667–673.
Wise, A., and Cobanoglu, M.C. (2016). Predicting Targeted Cancer Therapeutics.
Yang, W., Soares, J., Greninger, P., Edelman, E.J., Lightfoot, H., Forbes, S., Bindal, N., Beare, D., Smith, J.A., Thompson, I.R., et al. (2013). Genomics of Drug Sensitivity in Cancer (GDSC): a resource for therapeutic biomarker discovery in cancer cells. Nucleic Acids Res. 41, D955–D961.
Yu, P., and Lin, W. (2016). Single-cell Transcriptome Study as Big Data. Genomics Proteomics Bioinformatics 14, 21–30.
Zhu, L., Lei, J., Devlin, B., and Roeder, K. (2016). A Unified Statistical Framework for Single Cell and Bulk RNA Sequencing Data.
(2017). The AI revolution in science.