Postdoc Call
Why join us?
Electricity was widely known at the end of the 19th century. It was anticipated to transform the industry, and rightly so, yet this did not happen until the 1920s. The early factories were designed with steam engines in mind, with all the constraints that go with that technology. Electricity only achieved its impact when it was used to enable new designs and ideas that were
previously impossible. (Source: David, Am Econ Rev, 1990)
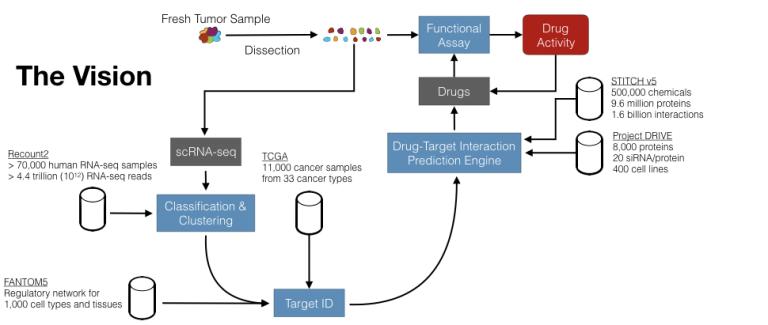
“Electrifying” drug discovery. We live in an age where machine learning is increasingly powerful, due to abundant computational power as well as algorithmic advances. Simultaneously, there is increasingly more biomedical data made available in public repositories. Finally, it is now possible to routinely characterize gene expression in single cells (scRNA-seq) thanks to recent technological advances. However, the cancer drug discovery paradigm mostly remains unchanged. The NCI-60, in the 1980s, ushered in a then-new paradigm of screening multiple (~60) cancer cell lines for drug activity to discover potential therapeutics. Since then, through automation and sheer force of will, larger screens have been conducted, with the latest containing about 1000 cell lines (Iorio et al., Cell, 2016). While helpful, the fundamental paradigm in this type of cancer drug discovery work is that cell lines in a dish represent cancer in the clinic. Unfortunately, that is not a good assumption: cancer is the therapeutic area with the lowest clinical success rate, with the likelihood of approval from phase I being only 5.1% in oncology, as opposed to 9.6% for all indications, or 19.1% for infectious diseases (Mullard, Nat Rev Drug Discov, 2016). Experts argue that the root cause for this oncology-specific problem is the suboptimal preclinical models used in cancer research (Hutchinson and Kirk, Nat Rev Clin Oncol, 2011). In our lab, our goal is to use machine learning, big data, and single-cell technologies to eventually build a human tumor-driven (and clinically relevant) cancer drug discovery pipeline.
What will you do?
You will build a generative probabilistic model of gene expression. Properly understanding RNA-sequencing data is imperative to our mission. We aim to build a generative probabilistic model of gene expression. Specifically, we will characterize gene expression with a mixture model of multiple cell type/state-specific and non-specific (i.e. shared) programs. We will estimate the contribution of each of these programs to an observed sample, and use that estimate to assign cellular type/state for well-characterized cell types/states (such as various tumor-infiltrating lymphocytes and their activation/exhaustion states). Likewise, we will use these estimates for clustering individual cells in the absence of clear cell type/state labels, as would be the case for malignant cells in the tumor microenvironment. In short, we will utilize this generative model for both clusters and classify single cells in scRNA-seq data. Simultaneously, the model will also provide insights into the genetic programs that are active in each cell. These insights will be akin to the gene set enrichment analysis (GSEA) results, which is a common analysis tool in biomedicine. Yet by simultaneous inference of the contribution of all programs, we seek to improve the quality of the results.
Advantages of doing this work with us. Single-cell RNA-seq is in the early stages and poised to be used with increasing frequency in the future. Thus, contributions to scRNA-seq data analysis can make a significant impact in the future, provided that the approaches are widely adopted. Being in a medical center, we think that we have a structural advantage in demonstrating the validity of our methods and therefore facilitating their wider adoption.
Required background. Advanced knowledge of statistical inference is necessary. Basic proficiency in programming is required. The biological background is not necessary, but certainly a plus — this is a medical center, and you can learn biology here.
Salary. UTSW Bioinformatics pays competitive postdoc salaries.
Contact
Interested candidates should contact the principal investigator via e-mail with their CV.
Murat Can Cobanoglu, Ph.D.
Email