To support the research projects in the Kraus Lab, we have developed and/or applied a wide variety of genomic tools, including novel computational pipelines designed to integrate, analyze, and visualize data from a wide variety of genomic (and proteomic) platforms. These include groHMM, a hidden Markov model-based algorithm for predicting primary transcription units based on GRO-seq data. We have used groHMM, which we deposited as an R-based package in Bioconductor for the community to use freely, to annotate thousands of previously unannotated noncoding RNA transcripts of unknown function. Furthermore, we have used genomic assays to examine the molecular mechanisms that drive signal-regulated transcriptional responses. These studies have characterized: (1) the robust and rapid changes that occur across the genome in response to estrogen and TNFα and (2) the expression of thousands of previously unannotated noncoding RNA transcripts, significantly altering our view of signal-regulated transcriptional responses.
We have recently developed TFSEE, a computational pipeline that integrates data from GRO-seq, RNA-seq, histone modification ChIP-seq, and motif searches, allowing for the simultaneous identification of putative subtype-specific enhancers and their cognate transcription factors. In addition to generating useful tools, our studies have helped to elucidate new facets of the genome and transcriptome.
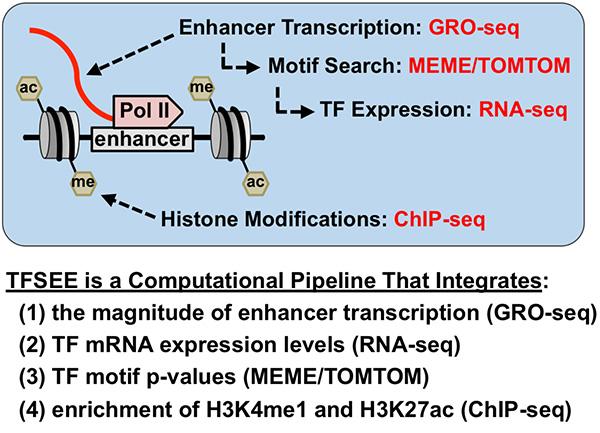
Selected Publications
Danko C.G., Chae M., Martins A., Kraus W.L. (2014) groHMM: GRO-seq Analysis Pipeline. R package version 1.0.0. Bioconductor (Software) www.bioconductor.org/packages/release/bioc/html/groHMM.html
Chae M, Danko CG, Kraus WL (2015) groHMM: a computational tool for identifying unannotated and cell type-specific transcription units from global run-on sequencing data. BMC Bioinformatics 16(222). PMCID: PMC4502638
Danko C.G., Hyland S.L., Core L.J., Martins A.L., Waters C.T., Lee H.W., Cheung V.G., Kraus W.L., Lis J.T., Siepel A. (2015) Identification of active transcriptional regulatory elements from GRO-seq data. Nature Methods 12(5):433-438. PMCID: 4507281
Nagari, A., Murakami, S., Malladi, V. S., & Kraus, W. L. (2017) Computational approaches for mining GRO-Seq data to identify and characterize active enhancers. Methods in Molecular Biology 1468:121-138. PMCID: PMC5522910
Franco, H. L., Nagari, A., Malladi, V. S., Li, W., Xi, Y., Richardson, D., Allton, K. L., Tanaka, K., Li, J., Murakami, S., Keyomarsi, K., Bedford, M. T., Shi, X., Li, W., Barton, M. C., Dent, S. Y. R., Kraus, W. L. (2018) Enhancer transcription reveals subtype-specific gene expression programs controlling breast cancer pathogenesis. Genome Research 28(2):159-170. PMID: 29273624
Ryu K.W., Nandu T., Kim J., Challa S., DeBerardinis R.J., Kraus W.L. (2018) Metabolic regulation of transcription through compartmentalized NAD+ biosynthesis. Science 360(6389):eaan5780. PMID: 29748257
Huang D., Camacho V.C., Setlem R., Ryu K.W., Parameswaran B., Gupta R.K., Kraus W.L. (2020) Functional Interplay between Histone H2B ADP-Ribosylation and Phosphorylation Controls Adipogenesis. Molecular Cell 79(6):934-939. PMID: 32822587
Palavalli Parsons L.H., Challa S., Gibson B.A., Nandu T., Stokes M.S., Huang D., Lea J.S., Kraus W.L. (2021) Identification of PARP-7 substrates reveals a role for MARylation in microtubule control in ovarian cancer cells. Elife 10:e60481. PMID: 33475085
Gupte R., Nandu T., Kraus W.L. (2021) Nuclear ADP-ribosylation drives IFNγ-dependent STAT1α enhancer formation in macrophages. Nature Communications 12(1):3931. PMID: 34168143
Challa S., Khulpateea B.R., Nandu T., Camacho C.V., Ryu K.W., Chen H., Peng Y., Lea J.S., Kraus W.L. (2021) Ribosome ADP-ribosylation inhibits translation and maintains proteostasis in cancers. Cell 184:4531-4546.e26. PMCID: PMC8380725
Huang D., Camacho C.V., Martire S., Nagari A., Setlem R., Gong X., Edwards A.D., Chiu S.P., Banaszynski L.A., Kraus W.L. (2022) Oncohistone Mutations Occur at Functional Sites of Regulatory ADP-Ribosylation. Cancer Research 82(13):2361-2377. PMID 35472077